Demand Forecasting in Supply Chain Analytics: Imagine you’re running a shop that sells clothes. Some days, you might have more customers than you expect, and you run out of popular items. Other days, you may have stocked too many clothes, and now they just sit there, taking up space and costing you money.
Demand forecasting helps you avoid both of these problems. It’s all about predicting how many customers will come in, what they will want to buy, and when they’ll want it. By using demand forecasting, you can plan to have just the right amount of stock at the right time, so you don’t waste money or disappoint customers.
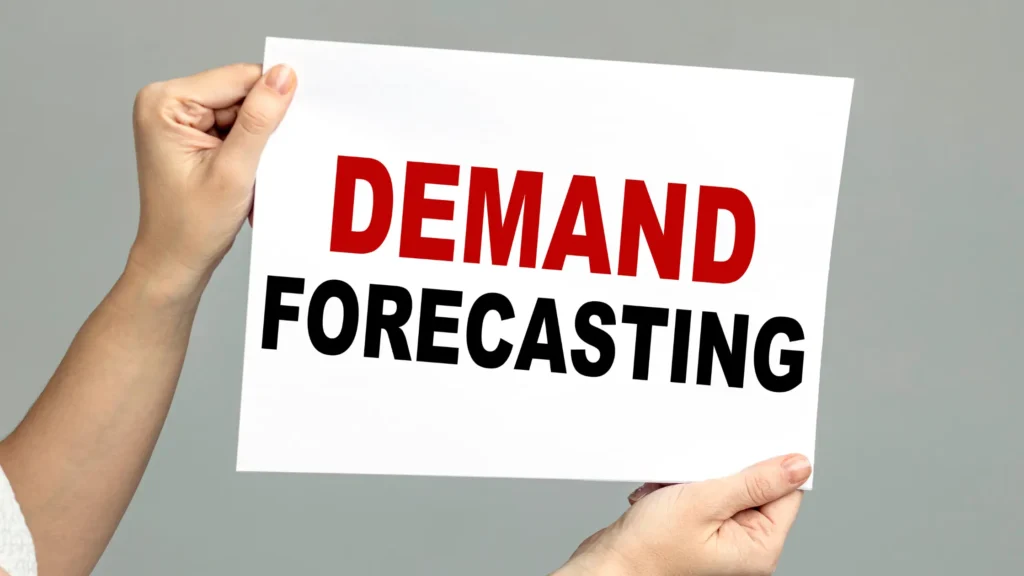
This idea isn’t just for a small shop—big companies, especially in supply chain management, use this to make sure their products are available when customers want them, whether they’re shopping online or at physical stores.
How Does Demand Forecasting Work in Real Life?
Now, demand forecasting is done using data, and here’s where it gets a bit technical. Companies have access to loads of information—past sales data, seasonality (like how sales go up before Christmas), economic conditions, and even things like social media trends. They use all this information to figure out what people are likely to buy in the future.
For example, if Nike wants to forecast how many shoes to produce next year, they won’t just guess. They’ll look at:
- Historical sales data: How many shoes were sold last year?
- Seasonal trends: Do more people buy sneakers during spring?
- External factors: Are there any major sports events happening that might spike interest?
By analyzing this data, they can predict with some accuracy how many shoes they’ll need to make, avoiding both overstocking (where they make too many shoes) and stockouts (where they don’t have enough to meet demand).
Different Types of Demand Forecasting Techniques
When it comes to demand forecasting in supply chain analytics, companies use different methods depending on what they’re trying to predict. Here are some of the most common:
- Time Series Analysis: This method looks at past data to predict the future. It works great if your demand is steady or follows a pattern over time, like winter coats always selling more in November and December.
- Moving Averages: This method looks at an average of sales data over a certain period to smooth out spikes and dips.
- Exponential Smoothing: Similar to moving averages, but it gives more importance to recent data. If you sold more shoes last week, this method assumes that’s a stronger indicator of future sales than what happened six months ago.
- Causal Models (Regression Analysis): Sometimes, demand is influenced by factors outside of your control, like the economy or weather. Causal models try to understand these relationships. For example, if it’s unusually rainy, maybe people will buy fewer sneakers and more boots. This method looks at how external factors (like the weather or a new ad campaign) can affect sales.
- Machine Learning: You’ve probably heard a lot about AI and machine learning. These tools are being used more and more in supply chain analytics. They can take in huge amounts of data, learn from it, and give even more accurate forecasts than traditional methods. Machine learning is like having a really smart assistant who can crunch massive amounts of data quickly and make adjustments in real time.
Why Do Companies Care About Demand Forecasting?
For big companies, forecasting demand correctly is like having a crystal ball—it saves them a ton of money and keeps customers happy. Let’s look at a few reasons why it’s so important:
- Reduces Inventory Costs: If a company makes too many products, they have to store them. Storing things costs money—warehouses, insurance, even the risk of items getting damaged or becoming obsolete. With demand forecasting, a company can make just the right amount of products and avoid overstocking.
- Keeps Customers Happy: Ever been to a store and found out the thing you wanted was sold out? Frustrating, right? Companies don’t want that. By forecasting demand, they can make sure the products people want are always available, improving customer satisfaction.
- Optimizes Production: Manufacturing is expensive. If a factory is running when there’s no need, it wastes money. On the flip side, if demand spikes and they weren’t prepared, it could cause delays. Demand forecasting helps companies plan their production schedules efficiently, ensuring that they’re always making just enough to meet demand.
- Helps with Supplier Relationships: Companies rely on suppliers to provide raw materials. If they can forecast demand accurately, they can give their suppliers enough time to prepare, ensuring smooth operations without delays. This also makes relationships with suppliers stronger.
Challenges in Demand Forecasting
While forecasting is incredibly useful, it’s not always easy. Here are a few challenges:
- Data Inaccuracy: If the data a company uses is wrong—say, if they don’t account for a major change in customer behavior—then their forecast will also be wrong. This is why companies need to clean and verify their data before making predictions.
- Demand Variability: Some industries, like fashion or technology, have highly unpredictable demand. Trends can change quickly, and something that was hot last month might be outdated now. These industries need more flexible and responsive forecasting models.
- Collaboration Between Teams: Demand forecasting requires input from many parts of a company—sales, marketing, finance, and production. If these teams aren’t working together and sharing information, forecasts might not be as accurate as they could be.
How Data Analytics Helps Improve Demand Forecasting
In today’s world, data analytics is making demand forecasting smarter. Companies use tools like Power BI, Tableau, and machine learning algorithms to analyze massive amounts of data, spot patterns, and adjust their forecasts in real time.
For example, if a company notices that sales of a certain product are unexpectedly rising due to a viral TikTok video, they can adjust their forecast immediately. This real-time data integration is crucial for businesses that operate in fast-changing markets.
Data visualization tools like Power BI also help companies see trends and make decisions faster. Instead of looking through spreadsheets, they can see visual representations of sales data and spot trends at a glance. This makes it easier for teams to communicate and act quickly.
Conclusion: Why You Should Care About Demand Forecasting
You might wonder why this matters to you. Well, understanding demand forecasting is a fundamental part of managing any business. Whether you’re selling products online, managing a retail store, or working in a massive company’s supply chain department, knowing how to predict and meet demand will always be valuable.
In a world that’s becoming more data-driven every day, learning how to analyze data and use it to make smart decisions is a crucial skill. And as you dive deeper into supply chain analytics, mastering demand forecasting is one of the best places to start.
So, the next time you buy something online, think about the process that went into making sure that item was in stock just when you needed it. That’s demand forecasting at work!
Key Takeaways:
- Demand forecasting is the process of predicting future demand using past data.
- It helps businesses reduce costs, improve customer satisfaction, and optimize production.
- There are different forecasting techniques, such as time series analysis, causal models, and machine learning.
- Data analytics tools make demand forecasting smarter and more responsive to market changes.