In today’s increasingly data-driven world, data analysis is a key driver of success in supply chain management. As businesses accumulate vast amounts of data from various sources, the ability to transform this raw data into actionable insights is crucial for maintaining a competitive edge. This article will explore the role of data analysis in supply chain management, highlight its importance, and discuss how companies can leverage it effectively.
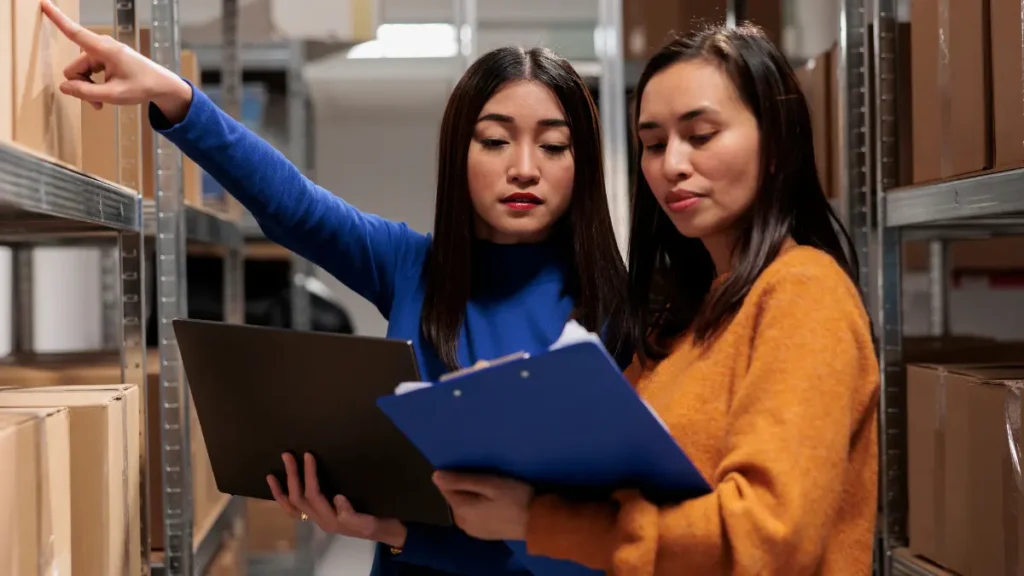
The Data Analysis Process
The data analysis process in supply chain management involves multiple stages, each designed to ensure the reliability and relevance of the data:
- Identifying Data: Pinpoint the required data and its sources, such as inventory levels, supplier performance, or customer demand.
- Cleaning Data: Remove duplicates, inconsistencies, or errors to ensure data accuracy.
- Transforming Data: Convert raw data into a usable format using techniques like aggregation and normalization.
- Modeling Data: Apply statistical or machine learning models to uncover trends and insights.
- Reporting & Visualization: Present findings through dashboards or reports to communicate actionable insights.
The Importance of Data Analysis in Supply Chain Management
Data analysis in supply chain management offers businesses several advantages:
- Customer Demand Insight: Understand customer preferences and forecast future demand based on historical data.
- Inventory Optimization: Evaluate stock levels to avoid overstocking or stockouts, ensuring smooth operations.
- Improved Forecasting: Predict future supply and demand needs, enabling companies to anticipate changes in the market.
- Data-Driven Decision-Making: Reduce reliance on intuition by making informed decisions based on real-time data.
Core Components of Supply Chain Analytics
Supply chain analytics can be categorized into the following components, each serving a unique purpose to enhance the overall performance of the supply chain.
1. Descriptive Analytics
- Purpose: Understand historical events.
- Example: A company might analyze the past six months’ data to determine trends in sales or inventory fluctuations. For instance, it could reveal a 15% drop in car sales, prompting further investigation.
2. Diagnostic Analytics
- Purpose: Explain why something happened.
- Example: Diagnostic analytics could help identify why there was a sudden increase in supply chain delays. In this scenario, it might reveal that disruptions were caused by fuel price hikes or logistical bottlenecks.
3. Predictive Analytics
- Purpose: Forecast future trends.
- Example: Predictive models can forecast that car sales will drop by an additional 10% next quarter if current conditions persist. Companies often use time series analysis and machine learning to make these predictions.
4. Prescriptive Analytics
- Purpose: Recommend future actions.
- Example: Prescriptive analytics can suggest optimal inventory levels or supply chain adjustments based on predictive data. For example, companies might reduce production or offer promotions to address anticipated declines in demand.
5. Cognitive Analytics
- Purpose: Leverage AI to improve decision-making.
- Example: Cognitive analytics uses artificial intelligence to learn continuously from data. It could analyze customer feedback and social media trends to predict consumer preferences, helping businesses adapt their supply chains accordingly.
Example of Data Analysis in Supply Chain Management
Let’s examine a practical scenario of how data analysis can improve supply chain efficiency. A retail business could use various analytics methods to refine its operations:
- Descriptive: Generate reports on past inventory performance.
- Diagnostic: Investigate causes of supply chain delays.
- Predictive: Forecast future inventory requirements based on past data.
- Prescriptive: Recommend optimal stock levels and ordering schedules.
- Cognitive: Analyze supplier feedback using AI to ensure timely deliveries.
Trusting Your Data
Ensure data trustworthiness by focusing on:
- Data Quality: Accuracy, completeness, and consistency.
- Data Sources: Reliability of sources.
- Data Governance: Effective data management policies.
The Growing Need for Supply Chain Data Analysts
With data growth, the demand for skilled data analysts in supply chains rises. Data analysts:
- Organize Information: Structure and clean data.
- Distill Insights: Extract meaningful insights.
- Communicate Findings: Present data clearly to stakeholders.
Conclusion
Data analysis unlocks the full potential of supply chain operations by converting raw data into valuable insights. With the growing complexity of supply chains, adopting a data-driven approach is no longer optional—it is essential for long-term success. By refining skills in data analysis and ensuring data trustworthiness, businesses can enhance their efficiency, reduce costs, and improve customer satisfaction.
Further Reads
Big data analytics in supply chain management: A state-of-the-art literature review – This article provides a comprehensive review of the role of big data analytics in supply chain management, highlighting key trends, technologies, and case studies.
The impact of big data analytics capabilities on supply chain performance – This study examines how big data analytics capabilities influence supply chain performance, discussing various analytical techniques and their applications in improving efficiency and decision-making.
Keywords: Data Analysis in Supply Chain Analytics, Core Components of Supply Chain Analytics