Introduction
In the fast-paced world of global commerce, Artificial Intelligence (AI) and Machine Learning (ML) are becoming pivotal in optimizing supply chain operations. By automating decision-making processes, forecasting demand more accurately, and improving overall efficiency, these technologies offer a competitive advantage to businesses. As companies adopt AI and ML, they streamline inventory management, optimize logistics, and reduce operational costs—all critical factors in today’s volatile markets.
In this blog, we’ll explore how AI and ML are transforming supply chain optimization, discuss real-world use cases, and highlight the benefits and challenges of adopting these technologies.
The Role of AI and Machine Learning in Supply Chain Optimization
AI and ML are no longer futuristic concepts. In supply chain management, they are already making a significant impact by enhancing data analysis, improving forecasting, and automating routine tasks. AI enables machines to simulate human intelligence, while ML leverages algorithms to learn from historical data and continuously improve predictions over time.
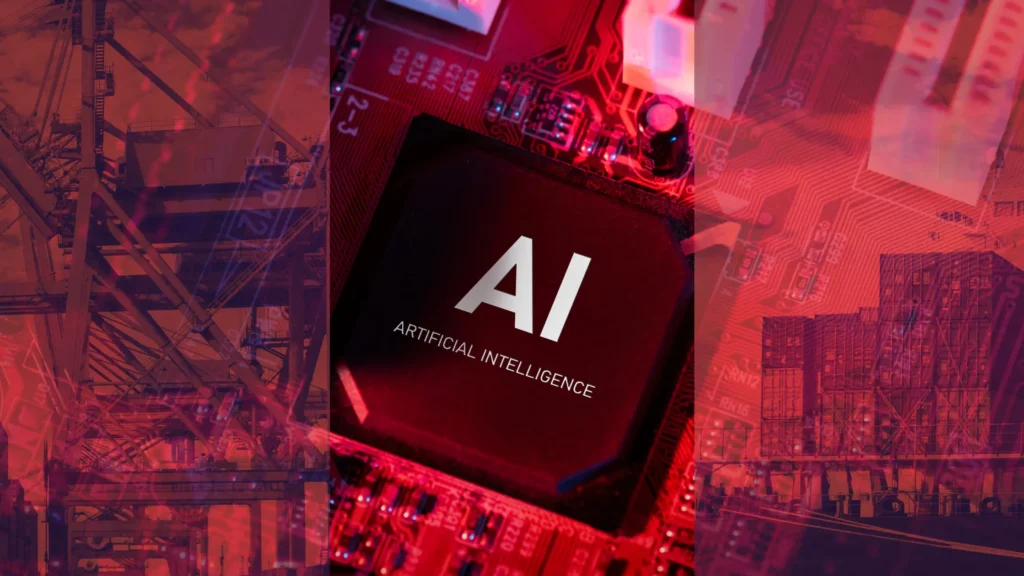
These technologies allow companies to process vast amounts of data quickly, providing insights that lead to better decision-making. Whether it’s predicting the right stock levels or optimizing delivery routes, AI and Machine Learning play a crucial role in supply chain optimization.
Key Applications of AI and Machine Learning in Supply Chain Management
1. Demand Forecasting
Accurately predicting customer demand is one of the most challenging aspects of supply chain management. Traditional forecasting models often fall short when unexpected external factors—such as economic shifts or weather conditions—affect demand. AI and ML provide more accurate forecasts by analyzing diverse datasets, including social media trends, market conditions, and even external factors like the weather.
Example: Amazon utilizes AI-driven demand forecasting to predict which products will be in demand, adjusting its inventory levels accordingly. By integrating external data such as weather patterns and shopping behaviors, Amazon reduces both overstocking and stockouts. This improves overall customer satisfaction while keeping costs low.
2. Inventory Optimization
Inventory management is crucial to ensure that products are available when and where they’re needed without overstocking or understocking. AI and ML models help predict the optimal time to reorder stock, taking into account variables such as lead times, historical sales data, and even market trends.
Case Study: Walmart uses AI to optimize its inventory levels across its global supply chain. By analyzing real-time sales data, Walmart adjusts inventory orders dynamically, ensuring that products are stocked in the right locations at the right times. This approach significantly reduces excess inventory and stockouts.
To read more about inventory management strategies, check out our post on Inventory Optimization in Supply Chain Analytics.
3. Logistics and Route Optimization
Efficient logistics are the backbone of supply chain operations, and AI can optimize delivery routes by factoring in real-time data such as traffic conditions, weather patterns, and delivery schedules. This not only reduces fuel consumption but also ensures timely deliveries, reducing shipping costs and enhancing customer experience.
Use Case: UPS employs the ORION system, which uses AI to optimize delivery routes. The system considers multiple factors, including traffic data and customer delivery windows, to calculate the most efficient delivery routes. This has allowed UPS to save millions of gallons of fuel each year.
Learn more about how the UPS ORION system works.
4. Supplier Relationship Management
Managing supplier relationships is another critical aspect of supply chain management. AI and ML can analyze supplier performance data, allowing businesses to identify potential risks or inefficiencies before they impact the supply chain. By tracking lead times, quality metrics, and delivery schedules, businesses can make informed decisions about their supplier relationships.
Example: Unilever uses AI to monitor its suppliers’ performance. This data-driven approach allows Unilever to assess which suppliers are delivering on time and maintaining high-quality standards, ultimately improving their procurement process and reducing risks.
For more insights on risk management in supply chains, explore our post on Navigating Conflicting Data Interpretations.
5. Predictive Maintenance
AI-driven predictive maintenance allows companies to monitor the health of their equipment in real time. By using IoT sensors, AI systems can predict when machines are likely to fail and schedule maintenance before a breakdown occurs, reducing downtime and saving on repair costs.
Case Study: Siemens uses predictive maintenance in its manufacturing operations, where AI algorithms analyze data from equipment to predict potential failures. This has reduced unplanned downtime by 30%, saving millions in operational costs.
Benefits of AI and Machine Learning in Supply Chain Optimization
Integrating AI and ML into supply chain management offers several key benefits:
- Improved Efficiency: AI can automate repetitive tasks like order processing, enabling employees to focus on more strategic areas of the business.
- Cost Savings: By optimizing routes, reducing stockouts, and cutting excess inventory, AI-driven supply chains can significantly lower operating costs. According to McKinsey, companies that use AI in their supply chains can reduce logistics costs by up to 15%. Read more about how AI can reduce costs on McKinsey’s website.
- Enhanced Accuracy: ML algorithms refine their predictions over time, improving the accuracy of demand forecasting and logistics planning.
- Increased Agility: AI-powered supply chains can adapt quickly to changes in market conditions or disruptions, ensuring they stay competitive in a dynamic environment.
Challenges of Implementing AI and Machine Learning in Supply Chains
While the benefits are clear, companies face several challenges when adopting AI and ML in their supply chain operations:
- Data Quality: AI systems depend on large datasets to function effectively. Poor-quality or incomplete data can limit the accuracy of AI-driven insights.
- High Implementation Costs: The initial setup, including upgrading infrastructure and hiring AI experts, can be costly. This is especially challenging for small and medium-sized businesses.
- Integration with Legacy Systems: Many companies still rely on outdated technologies that are not AI-compatible, making the transition more complex and expensive.
- Talent Shortage: There is a growing demand for AI and ML expertise, and businesses may struggle to find professionals with the required skills to implement and manage these systems effectively.
The Future of AI and Machine Learning in Supply Chain Optimization
As AI and ML technologies continue to evolve, their applications in supply chains will become even more advanced. For instance, the development of autonomous vehicles and AI-powered warehouses will significantly improve supply chain efficiency and reduce human error. Additionally, advancements in predictive analytics will allow businesses to anticipate supply chain disruptions and mitigate risks before they occur.
The future of AI in supply chain management will not only focus on improving operational efficiency but also on enhancing resilience, making supply chains better equipped to handle challenges like global pandemics and trade disruptions.
Conclusion
AI and Machine Learning are revolutionizing the way companies manage their supply chains. By enabling more accurate demand forecasts, optimizing inventory levels, and improving logistics operations, these technologies are paving the way for smarter, more efficient supply chains. Despite challenges like data quality and high implementation costs, the long-term benefits—such as reduced operational costs and increased agility—make AI and ML invaluable tools for companies looking to thrive in a rapidly changing market.
For further insights into optimizing your supply chain, check out our blog on Tools and Techniques for Inventory Analysis.