Introduction:
In today’s fast-paced business environment, data analytics in supply chain management is essential. Leveraging data analytics within SCM not only optimizes operations but also enhances decision-making. This blog delves into the critical role of data analytics in supply chain management(SCM), answering common questions and exploring its career potential and practical applications.
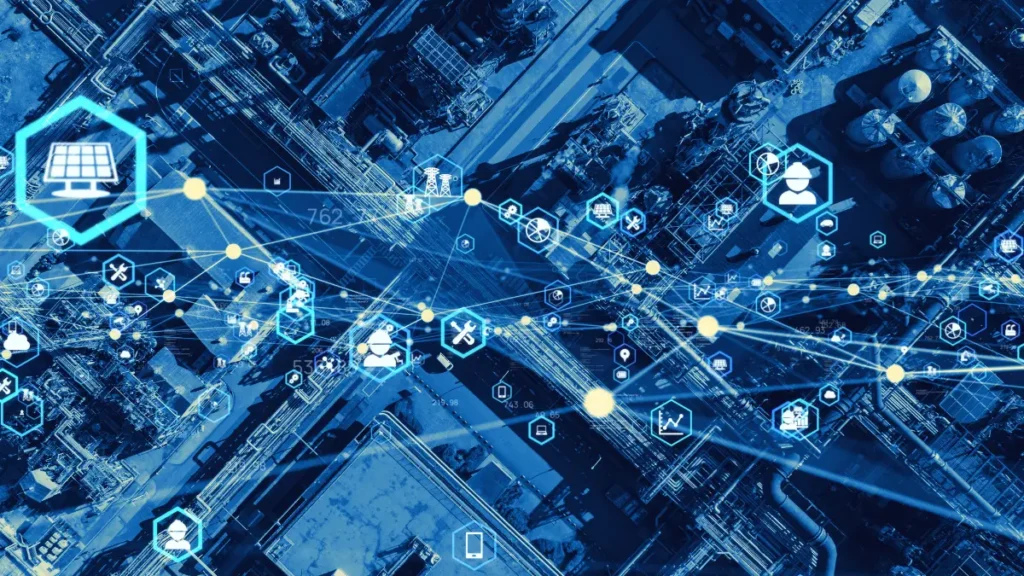
Understanding the Role of Data Analytics in SCM:
Use of data analytics in this domain involves the use of advanced analytical tools and techniques to interpret and utilize data from various stages of the supply chain. This helps organizations improve efficiency, reduce costs, and respond more effectively to market demands.
Is Data Analytics Used in SCM?
Absolutely! Data analytics is a game-changer in the field of Supply Chain Management(SCM). Companies use it to forecast demand, optimize inventory levels, and streamline logistics. By analyzing historical data and market trends, businesses can predict future demand more accurately, reducing the risk of overstocking or stockouts.
What Does a Supply Chain Data Analyst Do?
A supply chain data analyst plays a pivotal role in optimizing supply chain operations. They gather and analyze data from different parts of the supply chain, identify patterns, and provide actionable insights. This includes monitoring inventory levels, evaluating supplier performance, and identifying areas for cost reduction, all facilitated by data analytics in supply chain management.
Is Supply Chain Analytics a Good Career?
Yes, supply chain analytics is an excellent career choice. The demand for skilled professionals in Supply chain management data analytics is growing, driven by the increasing reliance on data-driven decision-making. Professionals in this field can expect competitive salaries and opportunities for advancement as businesses continue to prioritize efficient supply chain management.
What is Supply Chain Analytics with Example?
Data analytics in scm involves analyzing data to make informed decisions. For example, a company might use predictive analytics to forecast demand for a product during the holiday season. By analyzing past sales data, market trends, and consumer behavior, the company can ensure they have adequate stock to meet demand, avoiding potential lost sales or excess inventory.
Examples of Data Analytics in SCM Applications:
- Demand Forecasting: Using historical sales data to predict future demand and optimize inventory levels.
- Supplier Performance Analysis: Evaluating supplier performance based on delivery times, quality of goods, and cost, helping to identify the best suppliers.
- Logistics Optimization: Analyzing transportation data to optimize delivery routes, reducing shipping costs and delivery times.
Tools for Data Analytics in SCM:
Several tools aid for data analytics in scm, including:
- Tableau: For data visualization and analysis.
- Power BI: To create interactive data reports and dashboards.
- SAP Integrated Business Planning: For demand planning and inventory optimization.
Supply Chain Analytics vs. Supply Chain Management:
Supply Chain Management (SCM) encompasses the end-to-end process of planning, executing, and controlling the flow of goods and services from suppliers to customers. It includes demand forecasting, procurement, production planning, inventory management, and logistics.
Supply Chain Analytics (SCA) is the application of data analysis, statistical models, and technology to improve decision-making within the supply chain. It focuses on extracting insights from data to optimize various components of SCM.
Aspect | Traditional Supply Chain Management (SCM) | Supply Chain Analytics / Data-Driven Supply Chain Management (DDSCM) |
---|---|---|
Forecasting Accuracy | – Relies on historical data and manual inputs – Often leads to less accurate demand forecasts | – Utilizes predictive analytics and machine learning – Results in more precise demand predictions |
Inventory Management | – Dependent on periodic reviews and fixed safety stock levels – Potential for overstocking or stockouts | – Employs real-time data and dynamic inventory policies – Optimizes stock levels and reduces carrying costs |
Procurement Efficiency | – Supplier selection based on established relationships and cost considerations – Limited to cost considerations | – Uses data analytics to assess supplier performance comprehensively – Optimizes procurement processes – Reduces supplier risks |
Logistics Optimization | – Standard transportation modes and networks – Limited real-time visibility and optimization | – Advanced routing algorithms – Real-time tracking technologies – Reduces transportation costs – Improves delivery performance |
Conclusion:
Incorporating data analytics into supply chain management into business operations transforms how organizations operate, making them more efficient and competitive. Whether you’re considering a career in this field or looking to implement analytics in your business, understanding its benefits and applications is crucial. By leveraging data analytics in supply chain management, organizations can enhance their supply chain processes, driving growth and customer satisfaction.
Additional Resources:
- Supply Chain Management: An International Journal – The impact of order fill rate on customer satisfaction.
- Journal of Business Logistics – Advanced inventory systems and fill rate performance.
- Harvard Business Review – Order fulfillment in retail: Best practices and case studies.
- Taylor & Francis Online – Demand forecasting in supply chain: A review of techniques and applications.
- Logistics Management – Warehouse management systems: Improving efficiency and accuracy.